Artificial Intelligence in the pharmaceutical industry
It is no secret that the biopharmaceutical industry is under significant pressure to increase yield, increase product quality, and manage shrinking margins. All of this points to increasing operational efficiency which cannot be done without embarking on a Digital Transformation journey.
But, what does Digital Transformation mean, in reality? It goes beyond investing in systems, storage, data centers, and resources to manage it all. For life sciences manufacturers, digital transformation fundamentally means investing in processes and solutions that enable them to use their data to better extract knowledge and actionable insights from development processes. Digital Transformation starts with data. Fortunately, the manufacturing process generates massive amounts of data from operators, instruments, equipment, and systems. The challenge that manufacturers face is that they lack the enterprise-wide, scalable data analytics infrastructure needed to take advantage of this information. First, data is siloed and each system (MES, ERP, LIMS, etc) manages its own data without necessarily interacting with other systems. Secondly, even if the data is aggregated, manufacturers do not have a systematic way to take advantage of the power of the data. There’s simply no efficient way to cut through the noise, identify and analyze the pertinent data, and create actionable information that decision makers can use to improve manufacturing processes, at scale.
Fortunately, there’s a solution: Artificial Intelligence and Machine Learning, and manufacturers are taking advantage of these advanced applications now. We will explore three scenarios where AI and ML can help manufacturers meet their goals.
1. Continued Process Verification (CPV)
In a guideline published by the FDA, CPV is a mechanism to ensure the verification and improvement of a manufacturing process after the initial stages of process qualification and validation (ICH Q13, 2021; FDA Process Validation Guidance, 2011). It provides the process manager a degree of flexibility defined in the control strategy to govern the production unit and adapt a product recipe according to inherent process variability.
Where does AI come in? In one example, PDA is coordinating a worldwide initiative led by Aizon and participated by the Universitat Autònoma de Barcelona, the UMBC, pharmaceutical industries and consultant firms. In this project, data produced by fermentation of Pichia pastoris was used to develop a series of AI models that learned the relationship between Critical Process Parameters (CPPs) and Critical Quality Attributes (CQAs), which together identified the ideal operating conditions for producing optimal batches.
As a result, they could perform a multivariate outlier detection algorithm to isolate low-quality batches before the process had finished. From there, an AI model can recommend operator controlled actions to improve the outcome of the batch process.
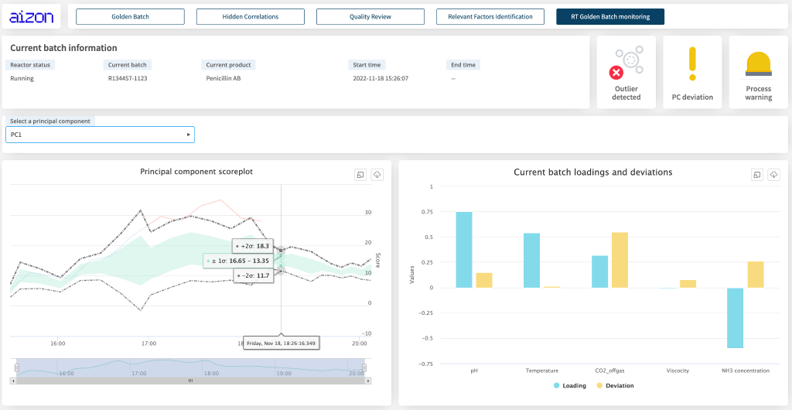
To continue reading this article you must login or register in the portal.
Para poder escribir un comentario debe iniciar sesión o darse de alta en el portal.